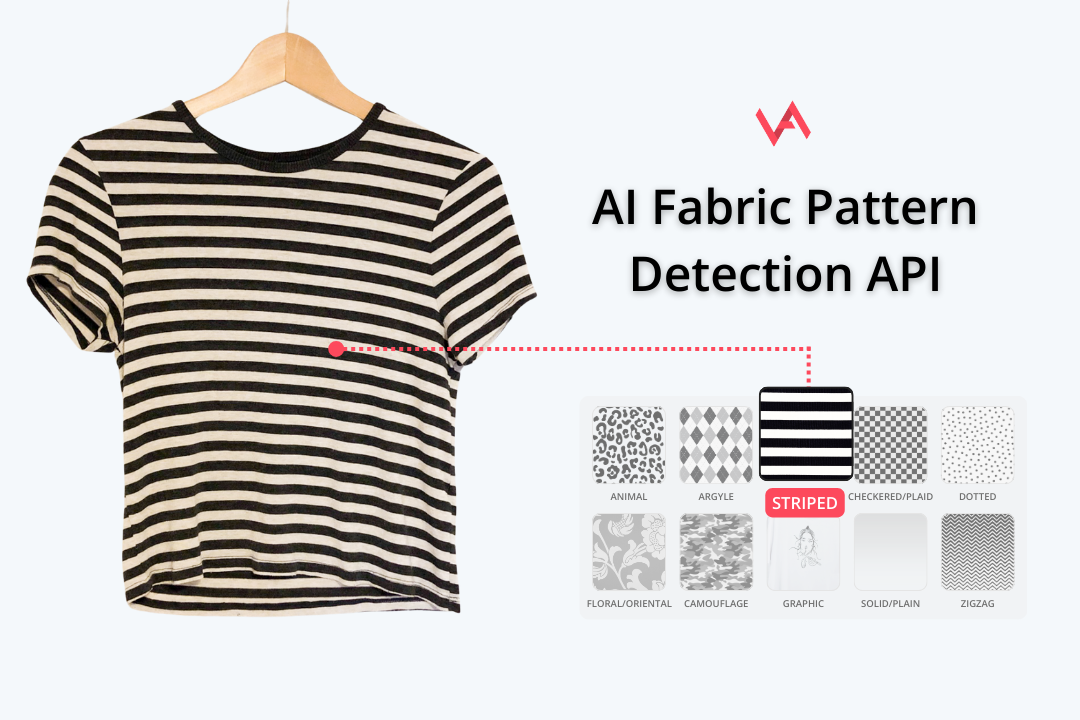
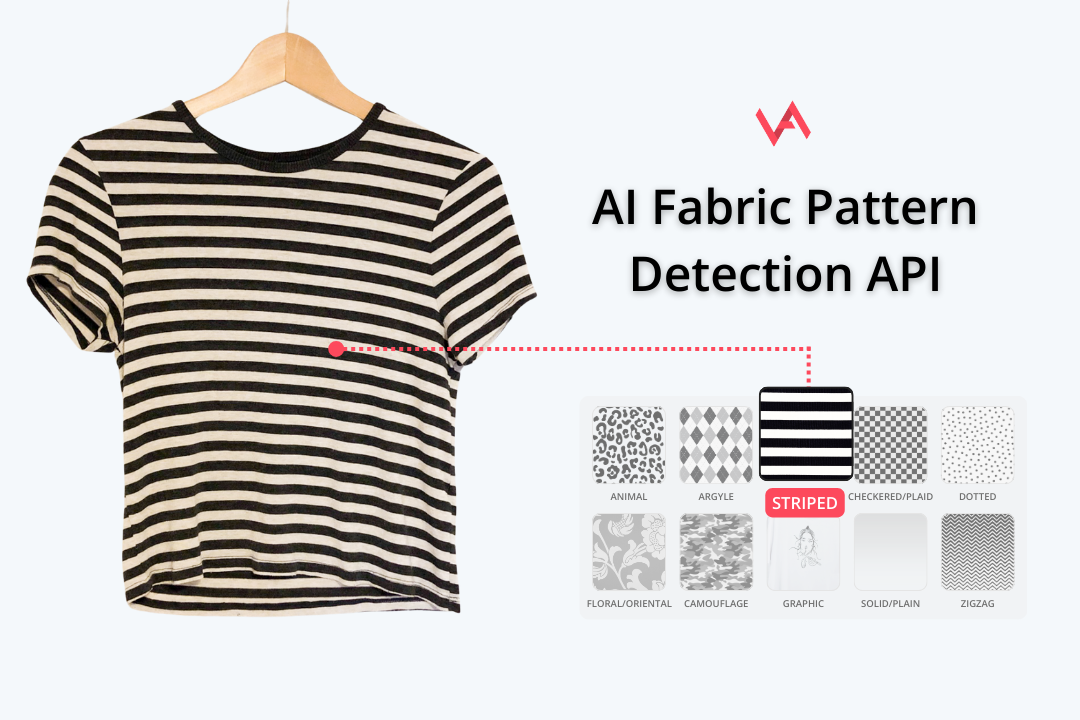
By Ivan Borko on November 2, 2020
The use of artificial intelligence and machine learning in the area of weather prediction is nothing new, but with the advent of deep learning and very large models, the number of use cases that can be solved with it is on the rise.
Area of weather prediction seems like a natural habitat for machine learning, because of the abundant data. Weather data like temperature, humidity, and rainfall rates have been collected for a long time (more than 100 years in some places) and it seems like an obvious direction for machine learning.
Satellite images of earth’s atmosphere are also available for quite some time.
One of the most respected global weather providers, the ECMWF, claims it produces 233 TBs of data every day.
The larger the machine learning model, the more complex tasks it can solve and the more data it needs. But Earth’s system is highly complex, especially these days when anthropogenic climate change brings faster weather changes and more extreme weather events.
Today’s best weather models are numerical models. They model atmospheric conditions with systems of non-linear differential equations with non-linear dependencies.
Solving these complex equations requires a lot of calculations and large supercomputers. They can be simplified if they are solved on smaller resolutions. That is why most global models output predictions on a 9km x 9km grid. Regional models tend to output 5km x 5km grids.
Although this simplifies the calculations, duration of one run is usually around 2-6 hours on a HPC (supercomputer). So, not only does it take a large and expensive infrastructure, it is also expensive to maintain and run several times a day.
This works fine if an area or a city is topographically simple, without high mountains or large green areas. For these, more complex cases, models can be biased or just less accurate.
Nowcasting is a weather prediction on a very short-term period, up to 3 hours (some resources define it as up to 2 hours, some up to 6). This blog will focus on short term rain prediction based on meteorological radars that record cloud reflectivity used to calculate rainfall rate.
Most of the global and regional numerical weather models predict rain (as well as other parameters in 1-hour or more often in 3 hour periods). As mentioned before, these predictions can take 2-6 hours to calculate.
Short term rainfall predictions are interesting for a number of industries. The agriculture sector can protect crops if a severe rain and possibly hail storm are approaching their area. Standard numerical models are often run on too small resolutions to enable this kind of prediction and don’t incorporate the latest weather data that is crucial for predicting such rapidly emerging phenomena.
Crop spraying shouldn’t be applied just before the rain, because the rain soon after application may wash the product from leaves and greatly reduce the level of protection or just rinse the product too deep into soil or flow to the water run-off.
Airports also use nowcasting data to reroute incoming traffic and guide aircraft around dangerous areas or clouds. Severe and local weather events can cause airport closure. Flight delays and cancellations are expensive in the short-term and, if the event is not well managed, also negatively impact the reputation of both airlines and airports.
Other industries can benefit as well: event organizers can postpone outside events if a rainstorm is approaching, open-air concert organizers can protect their equipment on time, etc.
Machine learning methods are being used for quite some time in some parts of numerical weather model pipelines, for example:
But the final goal of machine learning in weather forecasting is to build a tool that can use information on the current state of the atmosphere and predict the later time. Once trained, such a tool can be used to predict the future and be used as current numerical weather models.
Area of Deep learning has seen huge advancements over the last few years, and these days almost every computer vision task’s state-of-the-art is deep learning based. Deep means it uses deep neural networks, with a large number of layers. Such networks can take days to train on a number of GPUs, but once trained, inference takes usually less than a second.
It means that a deep learning based infrastructure can provide predictions within minutes of receiving new data. This makes it possible to always use the latest data available and provide fast-refreshing forecasts.
As deep learning is very hyped right now, it is influencing the development of hardware, cloud, GPUs and supercomputers.
Peter Dueben, ECMWF scientist working on numerical methods, states that:
“… machine learning is strongly influencing the development of future supercomputers. This means that the community of weather and climate modelling will need to learn in the near future how to make efficient use of such hardware optimised for very specific applications (requiring the use of dense linear algebra at very low numerical precision). “
One of the end-to-end prediction models is local rainfall prediction using radar maps. It can be easily formulated as a computer vision problem where based on a sequence of radar maps, a future map should be predicted.
A Chinese startup ColorfulClouds has specialized in a similar task - correcting the output of existing nowcasting systems such as NOAA which are not error free:
“Segmentation filters and removes false echoes from non-precipitation objects such as buildings, hills, birds and aircraft that may appear in the radar reflectivity images.“
While improving the numerical nowcast output is an interesting task, a team of researchers from Google has managed to completely replace the numerical nowcasting model using the U-Net model architecture. Their model beats three widely used models for weather nowcasting: Optical Flow, the Persistence model (repeat the last frame), and the High Resolution Rapid Refresh (HRRR) model by NOAA. Also, the deep learning approach is significantly faster and cheaper than the numerical methods which require supercomputers to operate.
Despite the promising results, using deep learning methods for precipitation nowcasting from radar maps has its limits. Although slow, numerical algorithms are significantly better at predicting weather at longer time horizons (6h+). Technically, this falls out of the nowcasting domain, but it also shows that historical data alone is not enough when it comes to long term weather forecasting. Whether deep learning methods will take over, or the combination of both numerical and deep learning methods will prevail, no one can tell but future.
AI and Machine Learning are penetrating more and more industries every year. Rapid model improvements have made ML the bright star from which we expect revolutionary advancements that can disrupt whole industries. In some cases, this might be true such as the autonomous vehicles industry, but for many, including the weather forecasting industry, the conclusion is still a bit blurry.
The only thing relatively certain is that ML performs very well when acting a supportive role in the classic processes. In terms of weather forecasting, this means we cannot expect ML models to completely replace all the current forecasting technology, but we have already seen that ML can help improve the existing numerical weather model.
We believe this trend is going to continue, but it does not exclude the slight possibility that one day, ML models could indeed completely replace the physics based weather model.
Have you learned something new from this article? If yes, share this article to spread the knowledge by clicking on one of the social media buttons below!
Are you interested in applying Machine Learning on your weather prediction use-case? Feel free to contact us and don’t forget to follow us for new updates!
Partner with us to develop an AI solution specifically tailored to your business.
Contact us